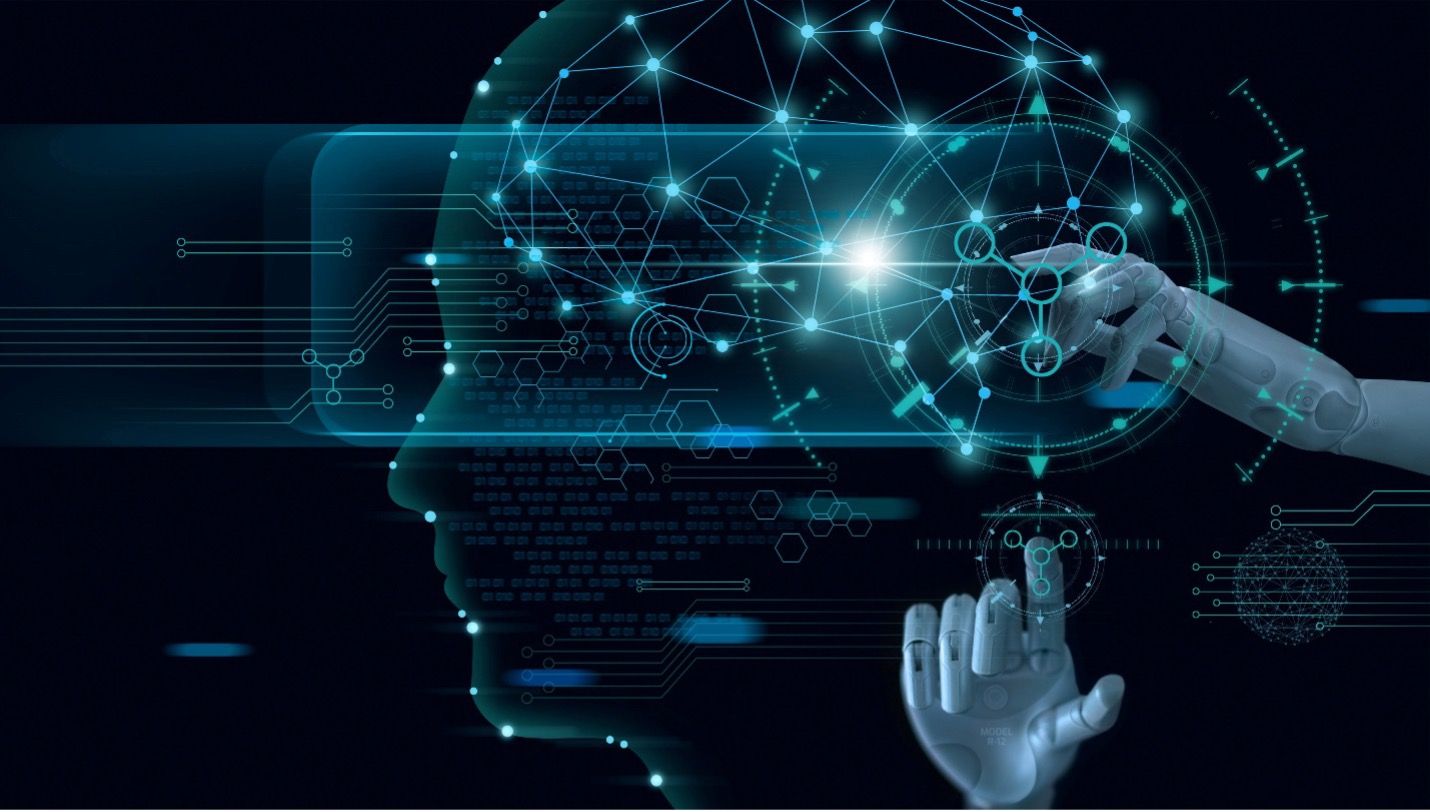
If you’re working in the business of modern technology, you’re likely to hear two terms associated with being keystones of data-driven decision-making: data science and decision science.
While both orbit around the central axis of data, they exhibit nuanced differences in their approaches and objectives. Let’s take a closer look at certain similarities and differences between these fields and shed some light on the essential skills they demand from practitioners.
The Convergence
At their core, both data science and decision science share a profound reliance on data. They embrace the notion that when harnessed effectively, data can illuminate a path to better decision-making. Both disciplines traverse the terrain of data collection, cleaning, and analysis in pursuit of meaningful insights. They stand as sentinels in problem-solving, employing analytical thinking to extract actionable intelligence from complex datasets.
Data scientist Matt Chapman wrote an article pointing out that the specialization of roles within data science is leading to a fragmentation of the field, with decision science offering a more holistic perspective on problem-solving and decision-making. It suggests that embracing decision science principles can enhance the effectiveness of data-driven approaches, particularly in corporate contexts.
Additionally, he says the rise of decision science offers more career options for data professionals, allowing them to specialize in areas that align with their interests and strengths.
Statistical Roots
A common thread that weaves through Data science and Decision science is the foundational importance of statistics. Concepts like regression analysis, hypothesis testing, and probability theory serve as the compass guiding their methodologies. These statistical underpinnings provide the tools necessary to navigate the vast sea of data and extract reliable insights.
Technological Tapestry
In both fields, proficiency in cutting-edge analytics tools and technologies is imperative. Whether it's wielding programming languages like Python and R or navigating platforms designed for data manipulation and visualization, a technological prowess forms the scaffolding upon which meaningful analyses are built.
Divergent Paths
Chris Dowsett used to work at Instagram and Spotify. He’s now the Vice President of Analytics and Data Science at hims & hers. Regarding the differences between data science and decision science, he points out that data scientists primarily focus on interpreting and analyzing data to enhance product development, emphasizing data quality and statistical rigor. In contrast, decision scientists center their analysis around specific business questions, aiming to provide actionable insights that aid decision-making.
So, while data science and decision science share a bedrock of data-driven methodology, they diverge in their focus and approach.
Data science trains its gaze on extracting insights and patterns from data. It embraces techniques like machine learning, deep learning, and predictive modeling to discern future trends from the tapestry of historical data.
In contrast, decision science casts a wider net. It encompasses not only the analysis of data, but also a deep dive into the psychology of decision-making. This includes the exploration of behavioral economics, cognitive psychology, and game theory, recognizing that a complex interplay of factors beyond the data often guides decisions.
Human-Centric Perspective
Here, decision science unfurls an additional layer of complexity. It places a significant emphasis on comprehending human behavior and cognitive processes. It unravels the biases, heuristics, and psychological undercurrents that shape decision-making. While not immune to these human elements, data science often centers its efforts on developing autonomous algorithms and models.
Temporal Perspectives
Data science and decision science also part ways in their considerations of time. Data science is often geared towards short- to medium-term forecasts, leveraging historical data to anticipate future trends. It excels in scenarios where patterns from the past can serve as a reliable compass for the future.
Decision science, on the other hand, adopts a more extended temporal perspective. It contemplates the potential impacts of decisions over prolonged periods, accounting for uncertainties, risks, and dynamic variables that may shape the outcomes.
Skills for Success in Both Fields
In the swiftly changing landscape of data-driven professions, Data science and Decision science success hinges on a mosaic of skills.
Advanced Analytics lies at the core. Proficiency in statistical techniques, machine learning algorithms, and predictive modeling forms the bedrock.
A Programming Competency is non-negotiable. Mastery of languages like Python, R, and SQL is imperative for wielding data as a tool for insight.
Data Visualization emerges as a critical skill, enabling the translation of complex information into digestible insights.
Domain Expertise lends context and relevance to data. Understanding the specific industry or domain allows for formulating strategies grounded in real-world nuances.
Ethical Considerations hold sway in both fields. Given the sensitivity of the information handled, a strong ethical compass ensures responsible and lawful use of data.
Finally, Effective Communication serves as the bridge between insights and action. The ability to convey findings and recommendations to non-technical stakeholders is paramount.
While bound by a common foundation in data analytics, data science and decision science embark on distinct journeys in pursuit of better decision-making. By recognizing the unique strengths of each field and nurturing a diverse skill set, professionals can position themselves at the forefront of the data-driven revolution. This empowers organizations to navigate the complexities of an increasingly data-rich and competitive landscape with confidence and clarity.
For data science bootcamp students, it's crucial to remember the evolving landscape of the field. Firstly, recognizing the emergence of decision science as a distinct discipline alongside data science is essential. Combining data science with behavioral sciences emphasizes the value of qualitative methods and holistic problem-solving. This underscores the importance of going beyond statistics and math and considering broader perspectives when approaching data-driven challenges.
Additionally, students should be aware of the increasing specialization within data science. This allows students to align their interests and strengths with specific areas within the data industry. Moreover, developing strong decision science skills will be crucial for asking pertinent questions and gaining meaningful insights from data as AI advances.
Staying adaptable and open to new roles and approaches within the data field will be critical for success.